Deep learning has had exciting progress in the last few years especially in supervised learning tasks in computer vision, language, speech, etc. The next decade will be focused on developing new techniques that will address its current shortcomings.
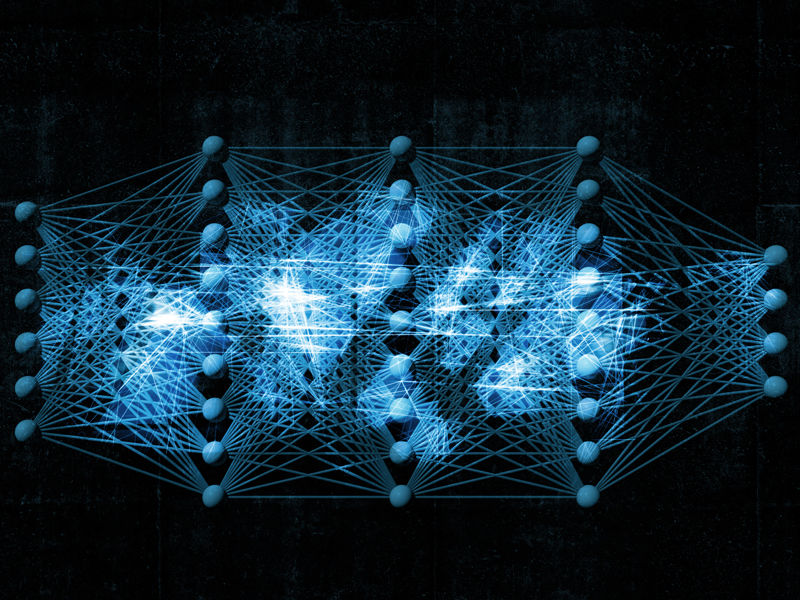
Here are some major areas that I see will be important in the next decade:
Labelled data is currently a big barrier to the adoption of deep learning. There will be more research in deep active learning and human-in-the-loop learning, where intelligent data collection is part of the machine-learning feedback loop. Self-supervision has shown recent promise for semi-supervised learning. We will see more development in these areas.
Controllable generative models of images, videos, text, and other data sources will be a major focus. Disentanglement of control inputs and extrapolation beyond the training data will be challenging and this cannot be accomplished through just memorization. Uncertainty quantification will be another important challenge. Current generative models like GANs do not provide good uncertainty estimates. Can we get both realistic data generation as well as accurate uncertainty estimates? This will be an important problem to tackle.
Hybrid deep learning models where deep learning is combined with other frameworks such as symbolic or causal reasoning will be investigated at scale. Incorporating domain knowledge and constraints into deep learning will be explored more. This is needed when there is limited data or when there are more challenging requirements for generalization. For instance, in robotics, infusing existing knowledge from physics and control algorithms into AI can help achieve safety and stability.
Synthetic data/simulations will be an important source for training data in data-limited applications like robotics and autonomous driving. Since simulations will not be perfectly accurate, algorithms will be needed for robust sim-to-real transfer and fine-tuning in the real domain.
In safety-critical applications such as autonomous systems guaranteeing reliability is a must. AI testing will mature and will draw tools from software testing, but we will also need innovations. Simulations provide a great platform for the failure testing of AI systems.
AI will move more to the edge. This will require aggressive (and sophisticated) model compression and real-time processing. Not all AI training will be done on the cloud as it is today. Some learning will move to the edge, especially when data privacy is a concern (federated learning). Machine learning in the wild, i.e. algorithms that quickly adapt to changes in data distribution and other environmental conditions will need to be developed further.
Comments