
Open AI’s analysis highlighted the major factors which are driving innovation in artificial intelligence today. Some of the key indicators of the trend are data, algorithmic advances and computing power. The non-profit AI research company’s latest analysis showcased that the amount of computing power used for training AI models has increased significantly – it has gone up to 3.5 month-doubling time, as compared to Moore’s Law 18-month doubling period.
Another key insight from the analysis is that even though algorithmic innovation and data is difficult to track, computing power, GPUs and TPUs, can be quantified. It therefore helps understand the measure of AI progress due to compute. This trend represents an increase by roughly a factor of 10 each year. This growth has largely been driven by custom hardware which allows more operations to be performed per second for a given price (GPUs and TPUs).
AI Computing Power Will Turn Into Three Way Race
This computing power led to many Deep Learning innovations — CNNs (Convolutional Neural Networks) and RNNs (Recurrent Neural Networks). The next wave of progress will come from Generative Adversarial Nets (GANs) and Reinforcement Learning, with some help thrown in from Question Answering Machines (QAMs) like IBM Watson. AI computing race will turn into a race of three:
High Performance Computing (HPC)
Neuromorphic Computing (NC)
Quantum Computing (QC)
On the other hand, chip maker Intel is betting big on probabilistic computing as a major component to AI that would allow future systems to comprehend and compute with uncertainties inherent in natural data and will allow researchers to build computers capable of understanding, predicting and decision-making. The core areas the company wants to address are — benchmark appli`cations, adversarial attack mitigations, probabilistic frameworks and software and hardware optimisation.
We see multiple reasons to believe that the trend could continue. Many hardware startups are developing AI-specific chips, some of which claim they will achieve a substantial increase in FLOPS/Watt (which is correlated to FLOPS/$) over the next 1-2 years. There may also be gains from simply reconfiguring hardware to do the same number of operations for less economic cost. On the parallelism side, many of the recent algorithmic innovations described above could in principle be combined multiplicatively — for example, architecture search and massively parallel SGD.
AI Chip Explosion
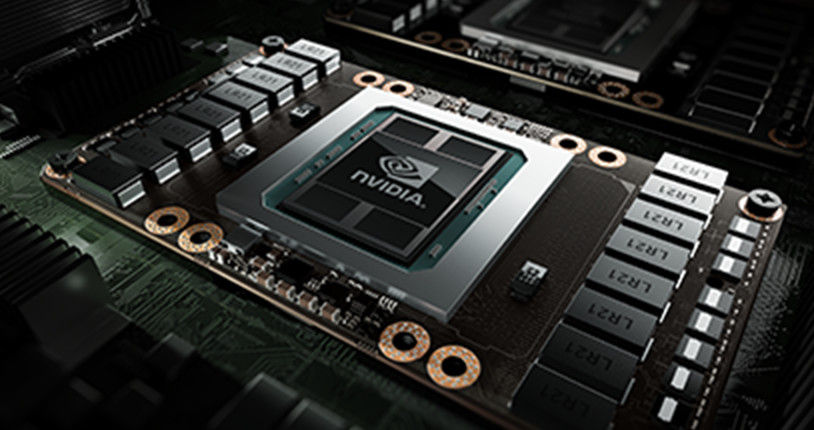
Today, every AI hardware startup and chip company is working on optimising high performance computing – the path is usually to stick to Deep Neural Net architectures and make it faster and easier to access. There may also be benefits realised from simply reconfiguring hardware to do the same number of operations at a reduced cost.
While Intel, Nvidia, and other traditional chip makers are working on capitalising on the new demand for GPUs, others like Google and Microsoft are busy developing proprietary chips of their own that make their own deep learning platforms a little faster. Google’s TensorFlow platform has emerged as the most powerful, general purpose solution backed up the proprietary chips, the TPU. Meanwhile, Microsoft is touting non-proprietary FPGAs while AI-focused hardware startups are working to make AI operations smoother. California-based Samba Nova Systems which is powering a new generation of computing by creating a new platform.
According to reports, this startup believes there is still room for disruption despite NVIDIA’s GPUs become the de facto standard for deep learning applications in the industry. The company which raised a $56 million funding in Round A wants to build a new generation of hardware that can work on any AI-focused device, be it a chip powering self-driving technology to even a server, news reports indicate. Other startups operating in a similar area are Graphcore and China’s Horizon Robotics which are also plowing investment in hardware and giving a stiff competition to GPUs — the backbone of all intensive computational applications for AI-related technologies.
Practically every large company from Facebook to Baidu invested in GPUs to fast-track their work around deep learning applications and train complex models. while in terms of efficiency, GPUs are pegged to be 10 times more efficient than CPUs, in terms of power consumption, NVIDIA claims GPUs are also driving energy efficiency in the computing industry.
Comments